Input Attention LSTM
This notebook shows how to generate interpretable results using deep learning model. The deep learning model used is Input Attention LSTM model of Qin et al., 2017. The dataset used is of Camels Australia
[2]:
# Some features used in this notebook are not available in the latest release of ai4water from pip which is 1.06
# at the moment. They will be available in ai4water's next release in 1.07. Since 1.07 is not currently available on
# pip, we will install ai4water from github using the following command.
# try:
# import ai4water
# except (ImportError, ModuleNotFoundError):
# !pip install git+https://github.com/AtrCheema/AI4Water.git@b0cb440f1c5e28477e2d1ea6f3ece2f68851bd93
[3]:
import tensorflow as tf
tf.compat.v1.disable_eager_execution()
D:\C\Anaconda3\envs\tfcpu27_py39\lib\site-packages\numpy\_distributor_init.py:30: UserWarning: loaded more than 1 DLL from .libs:
D:\C\Anaconda3\envs\tfcpu27_py39\lib\site-packages\numpy\.libs\libopenblas.EL2C6PLE4ZYW3ECEVIV3OXXGRN2NRFM2.gfortran-win_amd64.dll
D:\C\Anaconda3\envs\tfcpu27_py39\lib\site-packages\numpy\.libs\libopenblas.GK7GX5KEQ4F6UYO3P26ULGBQYHGQO7J4.gfortran-win_amd64.dll
warnings.warn("loaded more than 1 DLL from .libs:"
[4]:
import os
from easy_mpl import hist
import matplotlib.pyplot as plt
from ai4water import InputAttentionModel
from ai4water.datasets import CAMELS_AUS
from ai4water.postprocessing import Interpret
from ai4water.utils.utils import get_version_info
D:\C\Anaconda3\envs\tfcpu27_py39\lib\site-packages\sklearn\experimental\enable_hist_gradient_boosting.py:16: UserWarning: Since version 1.0, it is not needed to import enable_hist_gradient_boosting anymore. HistGradientBoostingClassifier and HistGradientBoostingRegressor are now stable and can be normally imported from sklearn.ensemble.
warnings.warn(
[5]:
for lib,ver in get_version_info().items():
print(lib, ver)
python 3.9.7 | packaged by conda-forge | (default, Sep 29 2021, 19:20:16) [MSC v.1916 64 bit (AMD64)]
os nt
ai4water 1.07
lightgbm 3.3.1
tcn 3.4.0
catboost 0.26
xgboost 1.5.0
easy_mpl 0.21.3
SeqMetrics 1.3.3
tensorflow 2.7.0
keras.api._v2.keras 2.7.0
numpy 1.21.0
pandas 1.3.4
matplotlib 3.4.3
h5py 3.5.0
sklearn 1.0.1
shapefile 2.3.0
fiona 1.8.22
xarray 0.20.1
netCDF4 1.5.7
optuna 2.10.1
skopt 0.9.0
hyperopt 0.2.7
plotly 5.3.1
lime NotDefined
seaborn 0.11.2
[6]:
dataset = CAMELS_AUS(
path="F:\\data\\CAMELS\\CAMELS_AUS" # path where data is downloaded. If data is not available, set path=None
)
inputs = ['et_morton_point_SILO',
'precipitation_AWAP',
'tmax_AWAP',
'tmin_AWAP',
'vprp_AWAP',
'rh_tmax_SILO',
'rh_tmin_SILO'
]
outputs = ['streamflow_MLd']
[10]:
data = dataset.fetch('401203', dynamic_features=inputs+outputs, as_dataframe=True)
data
[10]:
401203 | ||
---|---|---|
time | dynamic_features | |
1957-01-01 | et_morton_point_SILO | 8.062837 |
precipitation_AWAP | 0.000000 | |
tmax_AWAP | 20.784480 | |
tmin_AWAP | 4.358533 | |
vprp_AWAP | 8.142806 | |
... | ... | ... |
2014-12-31 | tmin_AWAP | 4.522077 |
vprp_AWAP | 8.885449 | |
rh_tmax_SILO | 26.714771 | |
rh_tmin_SILO | 75.013684 | |
streamflow_MLd | 322.791000 |
169472 rows × 1 columns
[11]:
data = data.unstack()
data.columns = [a[1] for a in data.columns.to_flat_index()]
data.head()
[11]:
et_morton_point_SILO | precipitation_AWAP | tmax_AWAP | tmin_AWAP | vprp_AWAP | rh_tmax_SILO | rh_tmin_SILO | streamflow_MLd | |
---|---|---|---|---|---|---|---|---|
time | ||||||||
1957-01-01 | 8.062837 | 0.0 | 20.784480 | 4.358533 | 8.142806 | 28.888577 | 88.900993 | 538.551 |
1957-01-02 | 8.519483 | 0.0 | 27.393169 | 4.835900 | 5.281136 | 23.516738 | 99.002080 | 531.094 |
1957-01-03 | 9.879688 | 0.0 | 28.945301 | 8.175408 | 12.920509 | 19.434872 | 77.429917 | 503.011 |
1957-01-04 | 6.744638 | 0.0 | 26.133843 | 7.017990 | 13.951027 | 42.350667 | 100.000000 | 484.512 |
1957-01-05 | 8.135359 | 0.0 | 21.450775 | 8.686258 | 12.168659 | 30.374862 | 87.634483 | 463.416 |
[12]:
data.shape
[12]:
(21184, 8)
[13]:
(data['streamflow_MLd'].values<0.0).sum()
[13]:
0
[14]:
(data['streamflow_MLd'].values==0.0).sum()
[14]:
0
[15]:
data.isna().sum()
[15]:
et_morton_point_SILO 0
precipitation_AWAP 0
tmax_AWAP 0
tmin_AWAP 0
vprp_AWAP 0
rh_tmax_SILO 0
rh_tmin_SILO 0
streamflow_MLd 0
dtype: int64
[16]:
_ = data.plot(subplots=True, sharex=True, figsize=(10, 10))
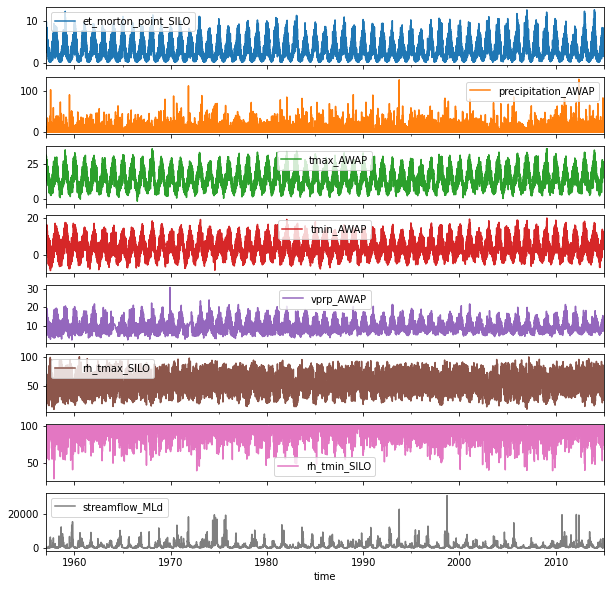
[17]:
_ = hist(data, share_axes=False, subplots_kws=dict(figsize=(12, 10)), edgecolor = "k", grid=False)
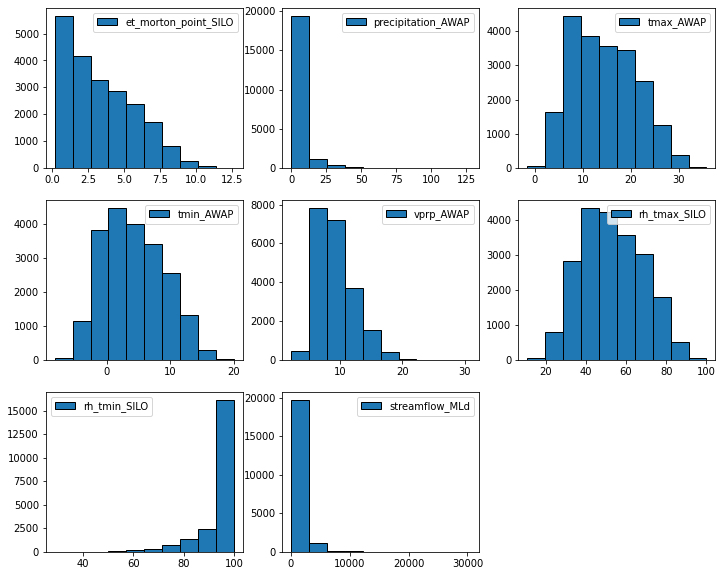
[18]:
skew_inputs = [
'precipitation_AWAP',
'rh_tmin_SILO'
]
[19]:
normal_inputs = ['et_morton_point_SILO',
'tmax_AWAP',
'tmin_AWAP',
'vprp_AWAP',
'rh_tmax_SILO',
]
[20]:
model = InputAttentionModel(
enc_config = {'n_h': 62, # length of hidden state m
'n_s': 62, # length of hidden state m
'm': 62, # length of hidden state m
'enc_lstm1_act': "elu",
'enc_lstm2_act': "relu",
},
input_features=inputs,
output_features=outputs,
epochs=500,
ts_args={'lookback':15},
lr=0.0049,
batch_size=16,
x_transformation=[{'method': 'robust', 'features': normal_inputs},
{'method': 'log', "replace_zeros": True, 'features': skew_inputs}],
y_transformation={'method': 'robust', 'features': outputs},
verbosity=0
)
building input attention model
[21]:
model.verbosity=1
[22]:
model.inputs
[22]:
[<tf.Tensor 'enc_input1:0' shape=(None, 15, 7) dtype=float32>,
<tf.Tensor 'enc_first_cell_state_1:0' shape=(None, 62) dtype=float32>,
<tf.Tensor 'enc_first_hidden_state_1:0' shape=(None, 62) dtype=float32>]
[23]:
model.outputs
[23]:
[<tf.Tensor 'dense/BiasAdd:0' shape=(None, 1) dtype=float32>]
[24]:
h = model.fit(data=data)
input_x shape: [(11854, 15, 7), (11854, 62), (11854, 62)]
target shape: (11854, 1)
inferred mode is regression. Ignore this message if the inferred mode is correct.
input_x shape: [(2964, 15, 7), (2964, 62), (2964, 62)]
target shape: (2964, 1)
Train on 11854 samples, validate on 2964 samples
Epoch 1/500
11840/11854 [============================>.] - ETA: 0s - loss: 0.8860
`Model.state_updates` will be removed in a future version. This property should not be used in TensorFlow 2.0, as `updates` are applied automatically.
11854/11854 [==============================] - 14s 1ms/sample - loss: 0.8859 - val_loss: 0.6613
Epoch 2/500
11854/11854 [==============================] - 6s 535us/sample - loss: 0.6698 - val_loss: 0.5784
Epoch 3/500
11854/11854 [==============================] - 6s 529us/sample - loss: 0.5649 - val_loss: 0.5355
Epoch 4/500
11854/11854 [==============================] - 6s 532us/sample - loss: 0.5270 - val_loss: 0.4771
Epoch 5/500
11854/11854 [==============================] - 6s 531us/sample - loss: 0.4819 - val_loss: 0.4564
Epoch 6/500
11854/11854 [==============================] - 6s 530us/sample - loss: 0.4615 - val_loss: 0.4084
Epoch 7/500
11854/11854 [==============================] - 6s 527us/sample - loss: 0.4076 - val_loss: 0.4688
Epoch 8/500
11854/11854 [==============================] - 6s 537us/sample - loss: 0.3892 - val_loss: 0.4032
Epoch 9/500
11854/11854 [==============================] - 6s 527us/sample - loss: 0.3519 - val_loss: 0.4458
Epoch 10/500
11854/11854 [==============================] - 6s 529us/sample - loss: 0.3585 - val_loss: 0.4269
Epoch 11/500
11854/11854 [==============================] - 6s 525us/sample - loss: 0.3369 - val_loss: 0.4451
Epoch 12/500
11854/11854 [==============================] - 6s 525us/sample - loss: 0.3166 - val_loss: 0.4580
Epoch 13/500
11854/11854 [==============================] - 6s 523us/sample - loss: 0.3213 - val_loss: 0.4300
Epoch 14/500
11854/11854 [==============================] - 6s 525us/sample - loss: 0.2915 - val_loss: 0.4084
Epoch 15/500
11854/11854 [==============================] - 7s 557us/sample - loss: 0.2741 - val_loss: 0.3997
Epoch 16/500
11854/11854 [==============================] - 6s 524us/sample - loss: 0.2666 - val_loss: 0.4371
Epoch 17/500
11854/11854 [==============================] - 6s 527us/sample - loss: 0.2748 - val_loss: 0.4323
Epoch 18/500
11854/11854 [==============================] - 6s 529us/sample - loss: 0.2599 - val_loss: 0.3841
Epoch 19/500
11854/11854 [==============================] - 6s 532us/sample - loss: 0.2283 - val_loss: 0.3901
Epoch 20/500
11854/11854 [==============================] - 6s 530us/sample - loss: 0.2249 - val_loss: 0.3655
Epoch 21/500
11854/11854 [==============================] - 6s 527us/sample - loss: 0.2213 - val_loss: 0.4015
Epoch 22/500
11854/11854 [==============================] - 6s 529us/sample - loss: 0.2080 - val_loss: 0.3814
Epoch 23/500
11854/11854 [==============================] - 6s 529us/sample - loss: 0.2048 - val_loss: 0.4125
Epoch 24/500
11854/11854 [==============================] - 6s 526us/sample - loss: 0.2009 - val_loss: 0.3687
Epoch 25/500
11854/11854 [==============================] - 6s 527us/sample - loss: 0.1784 - val_loss: 0.5114
Epoch 26/500
11854/11854 [==============================] - 6s 526us/sample - loss: 0.1840 - val_loss: 0.3700
Epoch 27/500
11854/11854 [==============================] - 7s 560us/sample - loss: 0.1833 - val_loss: 0.3600
Epoch 28/500
11854/11854 [==============================] - 6s 527us/sample - loss: 0.2156 - val_loss: 0.3684
Epoch 29/500
11854/11854 [==============================] - 6s 532us/sample - loss: 0.1686 - val_loss: 0.3616
Epoch 30/500
11854/11854 [==============================] - 6s 537us/sample - loss: 0.1480 - val_loss: 0.3747
Epoch 31/500
11854/11854 [==============================] - 6s 535us/sample - loss: 0.1410 - val_loss: 0.4073
Epoch 32/500
11854/11854 [==============================] - 6s 537us/sample - loss: 0.1536 - val_loss: 0.3528
Epoch 33/500
11854/11854 [==============================] - 6s 531us/sample - loss: 0.1628 - val_loss: 0.3771
Epoch 34/500
11854/11854 [==============================] - 6s 534us/sample - loss: 0.1488 - val_loss: 0.3711
Epoch 35/500
11854/11854 [==============================] - 6s 535us/sample - loss: 0.1443 - val_loss: 0.3875
Epoch 36/500
11854/11854 [==============================] - 6s 534us/sample - loss: 0.1280 - val_loss: 0.4221
Epoch 37/500
11854/11854 [==============================] - 7s 566us/sample - loss: 0.1536 - val_loss: 0.3384
Epoch 38/500
11854/11854 [==============================] - 6s 541us/sample - loss: 0.1279 - val_loss: 0.3258
Epoch 39/500
11854/11854 [==============================] - 6s 538us/sample - loss: 0.1332 - val_loss: 0.4067
Epoch 40/500
11854/11854 [==============================] - 6s 537us/sample - loss: 0.1333 - val_loss: 0.3578
Epoch 41/500
11854/11854 [==============================] - 6s 532us/sample - loss: 0.1152 - val_loss: 0.3633
Epoch 42/500
11854/11854 [==============================] - 6s 533us/sample - loss: 0.1113 - val_loss: 0.4077
Epoch 43/500
11854/11854 [==============================] - 6s 532us/sample - loss: 0.1202 - val_loss: 0.3671
Epoch 44/500
11854/11854 [==============================] - 6s 532us/sample - loss: 0.1155 - val_loss: 0.4412
Epoch 45/500
11854/11854 [==============================] - 6s 532us/sample - loss: 0.1246 - val_loss: 0.3626
Epoch 46/500
11854/11854 [==============================] - 6s 531us/sample - loss: 0.1062 - val_loss: 0.3785
Epoch 47/500
11854/11854 [==============================] - 6s 530us/sample - loss: 0.1128 - val_loss: 0.4564
Epoch 48/500
11854/11854 [==============================] - 7s 555us/sample - loss: 0.1083 - val_loss: 0.4337
Epoch 49/500
11854/11854 [==============================] - 6s 534us/sample - loss: 0.1072 - val_loss: 0.3948
Epoch 50/500
11854/11854 [==============================] - 6s 540us/sample - loss: 0.0985 - val_loss: 0.4036
Epoch 51/500
11854/11854 [==============================] - 6s 538us/sample - loss: 0.1065 - val_loss: 0.4161
Epoch 52/500
11854/11854 [==============================] - 6s 540us/sample - loss: 0.1038 - val_loss: 0.3724
Epoch 53/500
11854/11854 [==============================] - 6s 535us/sample - loss: 0.1031 - val_loss: 0.4129
Epoch 54/500
11854/11854 [==============================] - 6s 530us/sample - loss: 0.1029 - val_loss: 0.3731
Epoch 55/500
11854/11854 [==============================] - 6s 529us/sample - loss: 0.0895 - val_loss: 0.4002
Epoch 56/500
11854/11854 [==============================] - 6s 534us/sample - loss: 0.0882 - val_loss: 0.3604
Epoch 57/500
11854/11854 [==============================] - 6s 534us/sample - loss: 0.1000 - val_loss: 0.3837
Epoch 58/500
11854/11854 [==============================] - 6s 542us/sample - loss: 0.1255 - val_loss: 0.3730
Epoch 59/500
11854/11854 [==============================] - 6s 537us/sample - loss: 0.0982 - val_loss: 0.3623
Epoch 60/500
11854/11854 [==============================] - 6s 544us/sample - loss: 0.0777 - val_loss: 0.3528
Epoch 61/500
11854/11854 [==============================] - 6s 530us/sample - loss: 0.0867 - val_loss: 0.3903
Epoch 62/500
11854/11854 [==============================] - 6s 532us/sample - loss: 0.0851 - val_loss: 0.4092
Epoch 63/500
11854/11854 [==============================] - 6s 532us/sample - loss: 0.1017 - val_loss: 0.4183
Epoch 64/500
11854/11854 [==============================] - 6s 537us/sample - loss: 0.0841 - val_loss: 0.4137
Epoch 65/500
11854/11854 [==============================] - 6s 531us/sample - loss: 0.0982 - val_loss: 0.3605
Epoch 66/500
11854/11854 [==============================] - 6s 531us/sample - loss: 0.1120 - val_loss: 0.3662
Epoch 67/500
11854/11854 [==============================] - 6s 536us/sample - loss: 0.0717 - val_loss: 0.3686
Epoch 68/500
11854/11854 [==============================] - 6s 535us/sample - loss: 0.0679 - val_loss: 0.3970
Epoch 69/500
11854/11854 [==============================] - 6s 533us/sample - loss: 0.0946 - val_loss: 0.3725
Epoch 70/500
2368/11854 [====>.........................] - ETA: 4s - loss: 0.0926
---------------------------------------------------------------------------
KeyboardInterrupt Traceback (most recent call last)
~\AppData\Local\Temp\ipykernel_124924\414651862.py in <module>
----> 1 h = model.fit(data=data)
D:\mytools\AI4Water\ai4water\_main.py in fit(self, x, y, data, callbacks, **kwargs)
979 assert y is not None
980
--> 981 return self.call_fit(x=x, y=y, data=data, callbacks=callbacks, **kwargs)
982
983 def call_fit(self,
D:\mytools\AI4Water\ai4water\_main.py in call_fit(self, x, y, data, callbacks, **kwargs)
1016 if self.category == "DL":
1017
-> 1018 history = self._fit(inputs,
1019 outputs,
1020 callbacks=callbacks,
D:\mytools\AI4Water\ai4water\_main.py in _fit(self, inputs, outputs, validation_data, validation_steps, callbacks, **kwargs)
737 _kwargs.pop(k)
738
--> 739 self._call_fit_fn(
740 **_kwargs,
741 **kwargs,
D:\mytools\AI4Water\ai4water\_main.py in _call_fit_fn(self, x, **kwargs)
658 self._model.test_step = MethodType(test_step, self._model)
659
--> 660 return self.fit_fn(x, **kwargs)
661
662 def _fit(self,
D:\C\Anaconda3\envs\tfcpu27_py39\lib\site-packages\keras\engine\training_v1.py in fit(self, x, y, batch_size, epochs, verbose, callbacks, validation_split, validation_data, shuffle, class_weight, sample_weight, initial_epoch, steps_per_epoch, validation_steps, validation_freq, max_queue_size, workers, use_multiprocessing, **kwargs)
775
776 func = self._select_training_loop(x)
--> 777 return func.fit(
778 self,
779 x=x,
D:\C\Anaconda3\envs\tfcpu27_py39\lib\site-packages\keras\engine\training_arrays_v1.py in fit(self, model, x, y, batch_size, epochs, verbose, callbacks, validation_split, validation_data, shuffle, class_weight, sample_weight, initial_epoch, steps_per_epoch, validation_steps, validation_freq, **kwargs)
638 val_x, val_y, val_sample_weights = None, None, None
639
--> 640 return fit_loop(
641 model,
642 inputs=x,
D:\C\Anaconda3\envs\tfcpu27_py39\lib\site-packages\keras\engine\training_arrays_v1.py in model_iteration(model, inputs, targets, sample_weights, batch_size, epochs, verbose, callbacks, val_inputs, val_targets, val_sample_weights, shuffle, initial_epoch, steps_per_epoch, validation_steps, validation_freq, mode, validation_in_fit, prepared_feed_values_from_dataset, steps_name, **kwargs)
374
375 # Get outputs.
--> 376 batch_outs = f(ins_batch)
377 if not isinstance(batch_outs, list):
378 batch_outs = [batch_outs]
D:\C\Anaconda3\envs\tfcpu27_py39\lib\site-packages\keras\backend.py in __call__(self, inputs)
4184 self._make_callable(feed_arrays, feed_symbols, symbol_vals, session)
4185
-> 4186 fetched = self._callable_fn(*array_vals,
4187 run_metadata=self.run_metadata)
4188 self._call_fetch_callbacks(fetched[-len(self._fetches):])
D:\C\Anaconda3\envs\tfcpu27_py39\lib\site-packages\tensorflow\python\client\session.py in __call__(self, *args, **kwargs)
1481 try:
1482 run_metadata_ptr = tf_session.TF_NewBuffer() if run_metadata else None
-> 1483 ret = tf_session.TF_SessionRunCallable(self._session._session,
1484 self._handle, args,
1485 run_metadata_ptr)
KeyboardInterrupt:
Making predictions on training data
[26]:
_ = model.predict_on_training_data(data=data, plots=["regression", "residual", "prediction"])
input_x shape: [(11854, 15, 7), (11854, 62), (11854, 62)]
target shape: (11854, 1)
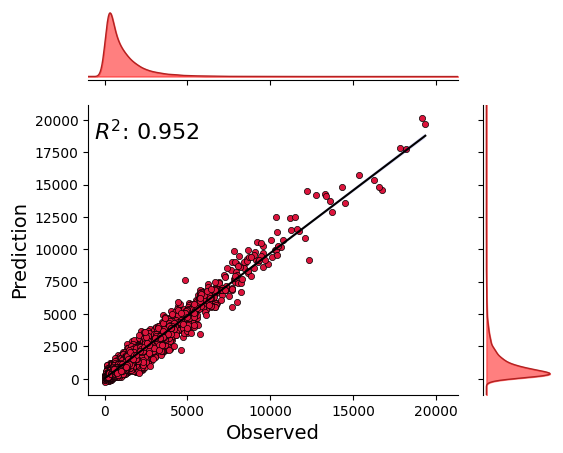
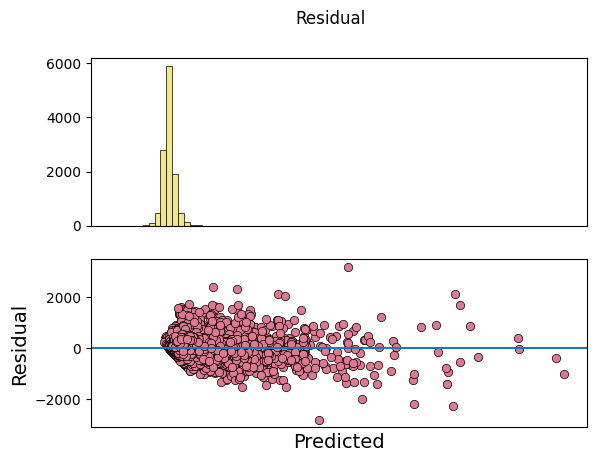
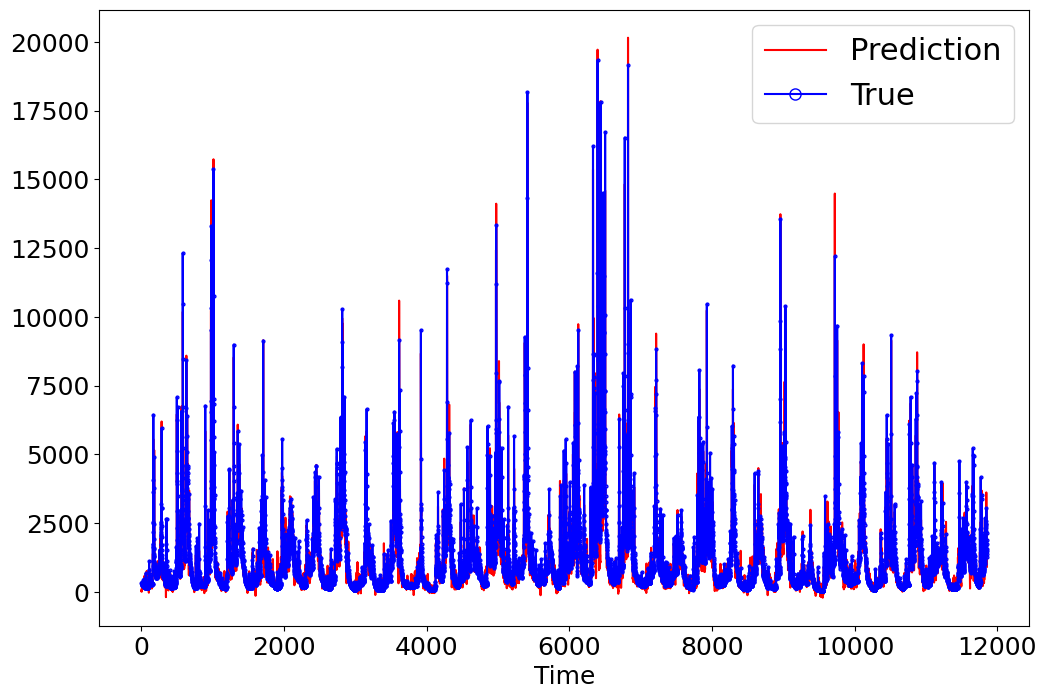
[27]:
model.evaluate_on_training_data(data=data, metrics="nse")
input_x shape: [(11854, 15, 7), (11854, 62), (11854, 62)]
target shape: (11854, 1)
argument test is deprecated and will be removed in future. Please
use 'predict_on_test_data' method instead.
[27]:
0.9517542124227427
Making predictions on test data
[28]:
_ = model.predict_on_test_data(data=data, plots=["regression", "residual", "prediction"])
input_x shape: [(6352, 15, 7), (6352, 62), (6352, 62)]
target shape: (6352, 1)
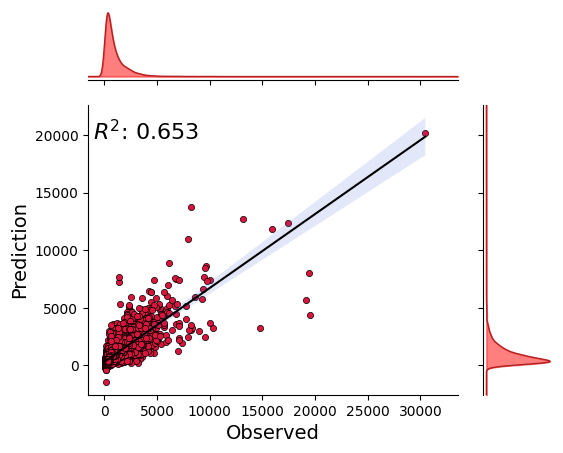
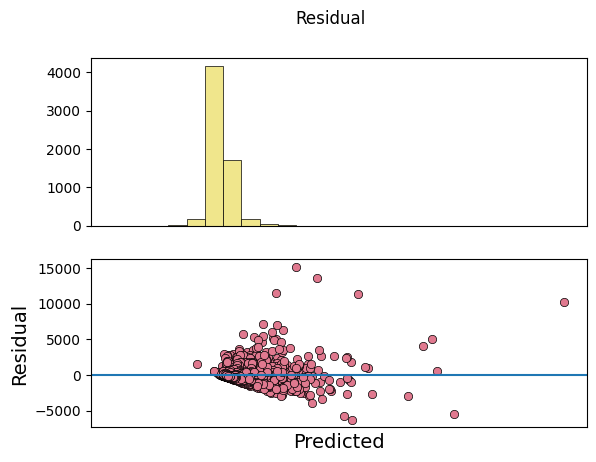
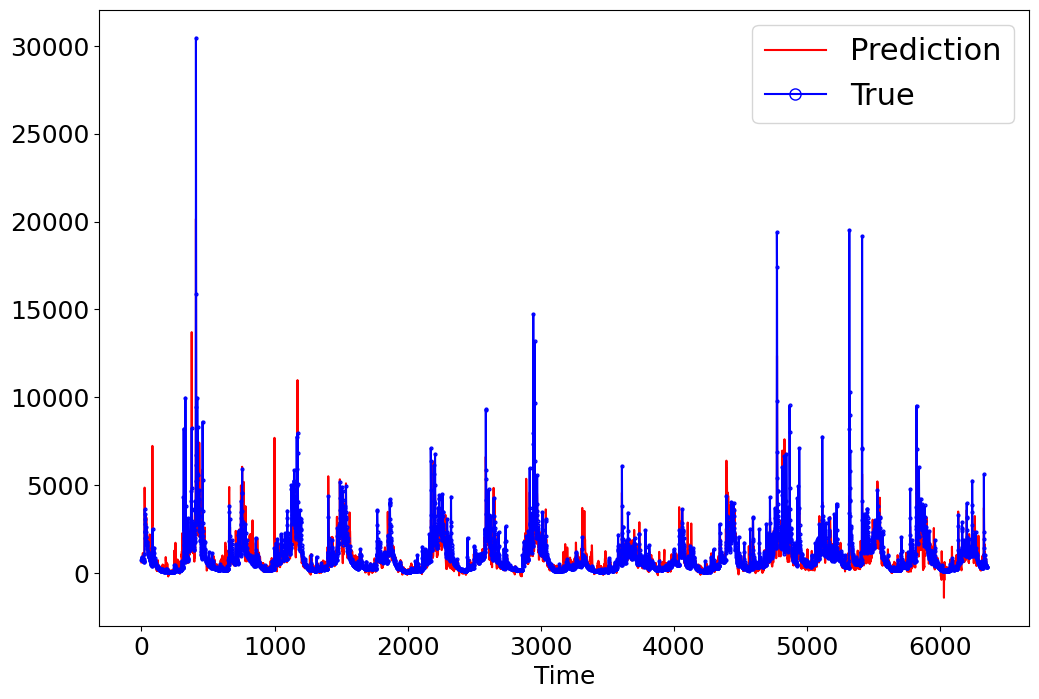
[29]:
model.evaluate_on_test_data(data=data, metrics=["nse", "r2", "rmse"])
input_x shape: [(6352, 15, 7), (6352, 62), (6352, 62)]
target shape: (6352, 1)
argument test is deprecated and will be removed in future. Please
use 'predict_on_test_data' method instead.
[29]:
{'nse': 0.6512428046837175, 'r2': 0.6530917330311746, 'rmse': 741.789854001792}
Getting interpretable results
[30]:
_ = model.plot_avg_attentions_along_inputs(data=data,
colorbar=True)
input_x shape: [(11854, 15, 7), (11854, 62), (11854, 62)]
target shape: (11854, 1)
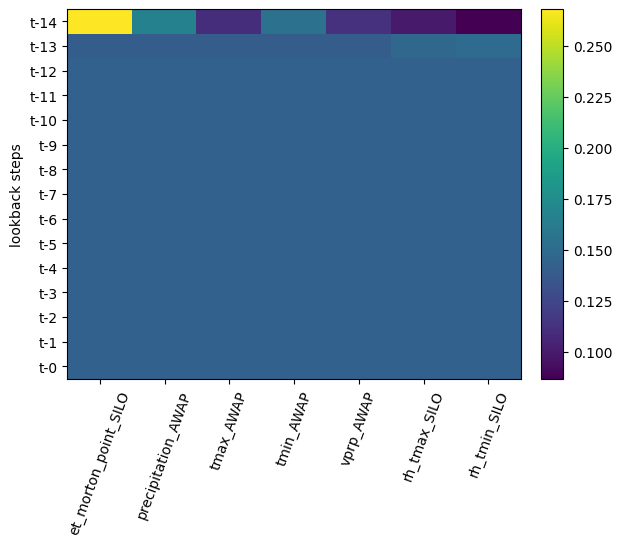
[31]:
x, y = model.test_data(data=data)
input_x shape: [(6352, 15, 7), (6352, 62), (6352, 62)]
target shape: (6352, 1)
[32]:
_ = model.plot_act_along_inputs(data=data,
feature="precipitation_AWAP",
cbar_params={"pad": 0.5}, cmap="jet")
input_x shape: [(11854, 15, 7), (11854, 62), (11854, 62)]
target shape: (11854, 1)
input_x shape: [(11854, 15, 7), (11854, 62), (11854, 62)]
target shape: (11854, 1)
argument test is deprecated and will be removed in future. Please
use 'predict_on_test_data' method instead.
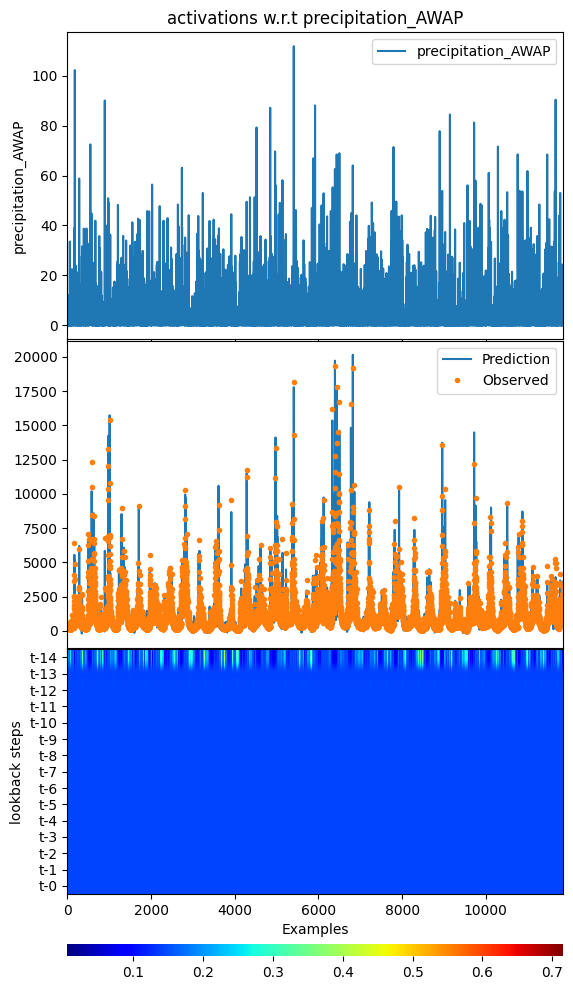
[33]:
_ = model.plot_act_along_inputs(data=data, feature="et_morton_point_SILO",
cbar_params={"pad": 0.5}, cmap="jet")
input_x shape: [(11854, 15, 7), (11854, 62), (11854, 62)]
target shape: (11854, 1)
input_x shape: [(11854, 15, 7), (11854, 62), (11854, 62)]
target shape: (11854, 1)
argument test is deprecated and will be removed in future. Please
use 'predict_on_test_data' method instead.
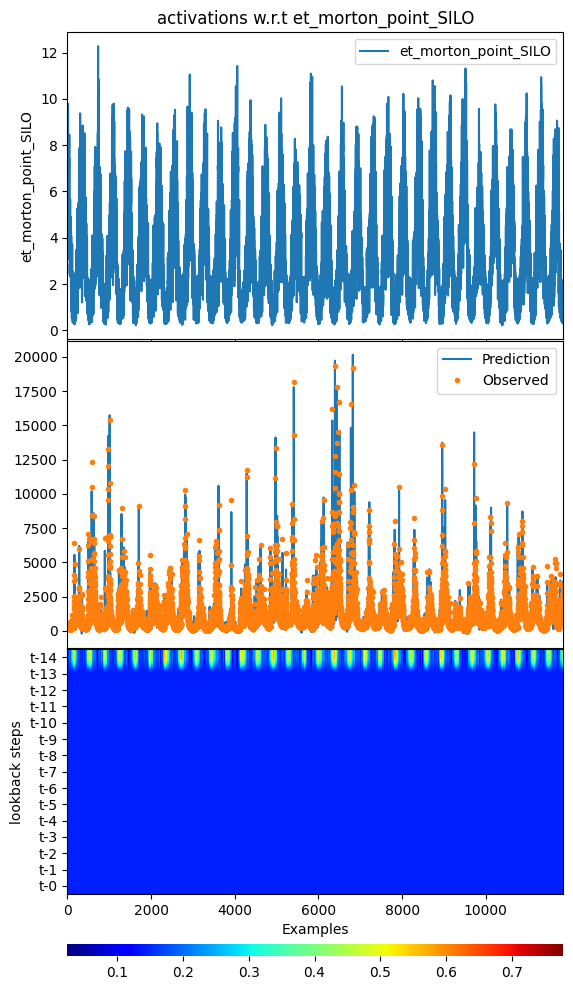
[34]:
_ = model.plot_act_along_inputs(data=data, feature="tmax_AWAP",
cbar_params={"pad": 0.5}, cmap="jet")
input_x shape: [(11854, 15, 7), (11854, 62), (11854, 62)]
target shape: (11854, 1)
input_x shape: [(11854, 15, 7), (11854, 62), (11854, 62)]
target shape: (11854, 1)
argument test is deprecated and will be removed in future. Please
use 'predict_on_test_data' method instead.
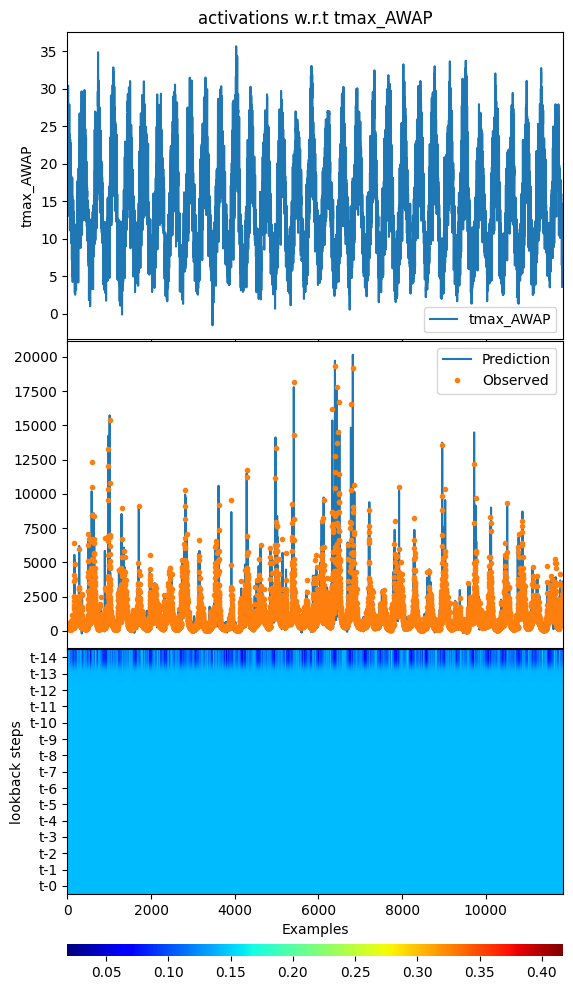
[35]:
print(f'All results are saved in {model.path}')
All results are saved in D:\mytools\ai4water_examples\docs\source\_notebooks\model\results\20230222_191908
[ ]: